AI-Powered Risk Based Testing
Instead of running the full test-suite, Appsurify selects and runs just the Tests impacted by recent developer changes via Proprietary AI Test Selection Technology. How?
In 3 simple steps:
- Auto-Map Tests to Code.
- See Changes to Code via Commit metadata.
- Auto-Execute only Tests impacted by Developer Changes.
Catch Bugs Earlier by Executing only the Tests relevant to Developer Changes in your CI/CD for instant Test feedback, optimized CI/CD Builds, and Infrastructure Savings.
Supports all Test frameworks, such as E2E, UI, Integration, Unit, etc.
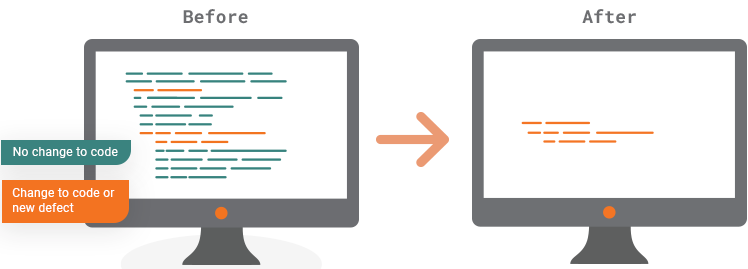
Reduce Infrastructure and Cloud Costs
Running Full Test Suite
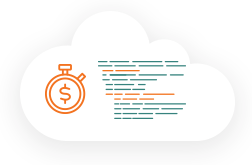

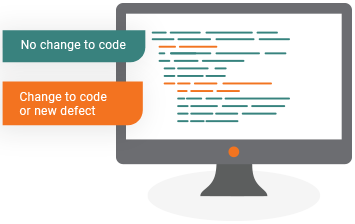
Test only what Matters
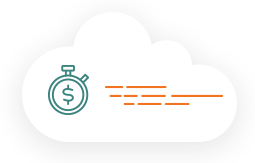

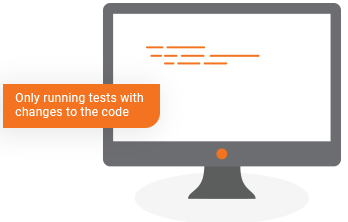
Save Time & Money running only Tests that Matter
Flaky Failures
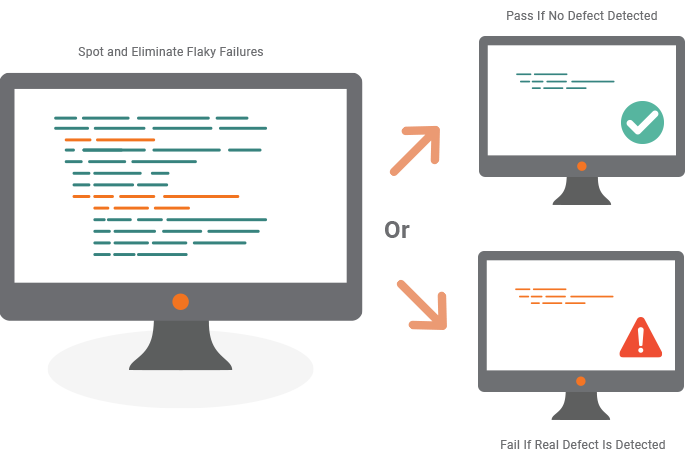
Flaky Test Failures
Automatically determine and quarantine a flaky test to pass the build on time and with confidence.
Appsurify can be configured to pass Tests when they’re Flaky and Fail them when they’re related to a Real Defect.
Eliminate the time consuming process of managing large unstable automation test suites and free up considerable time that would otherwise be wasted diagnosing random failures.
Troubleshoot only real Failures!
Supporting the Test Leaders
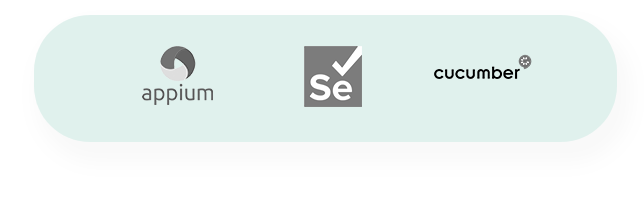
Appium
Selenium
Cucumber

AI-Powered Risk Based Testing

Flaky Test Failures

Risk Alerts
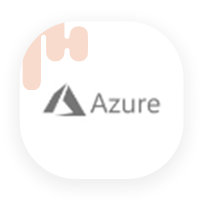
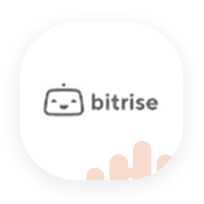
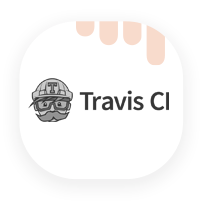
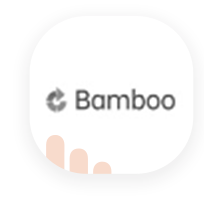
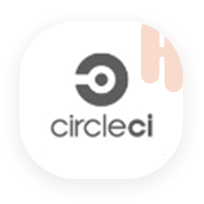
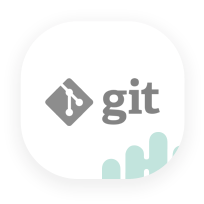
CI/CD
Repository
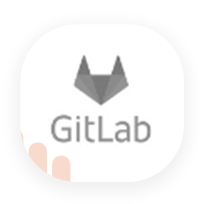
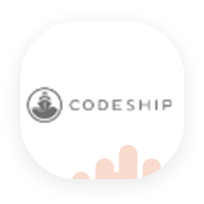
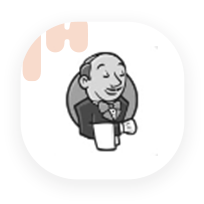
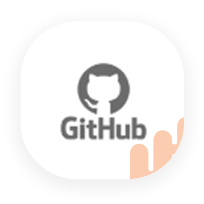
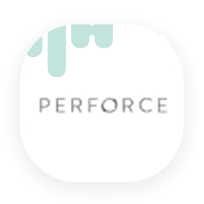
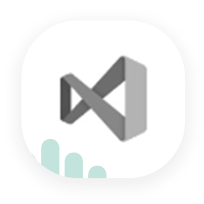
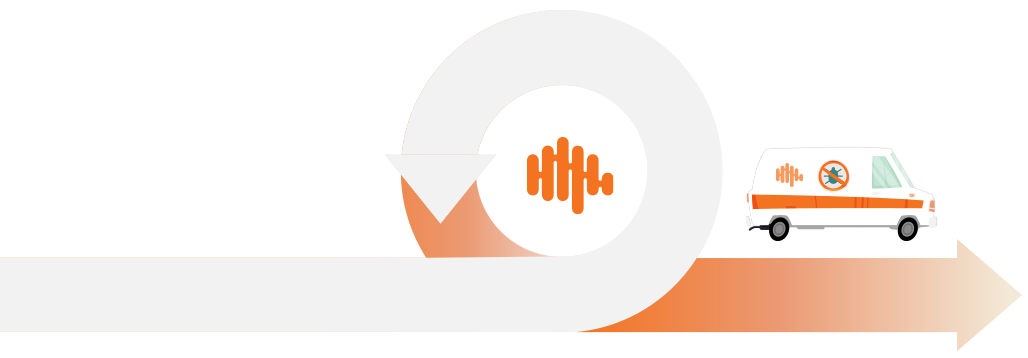